Initial LDA Instructions
User Manual:
Open the PDF directly: View PDF .
Page Count: 2
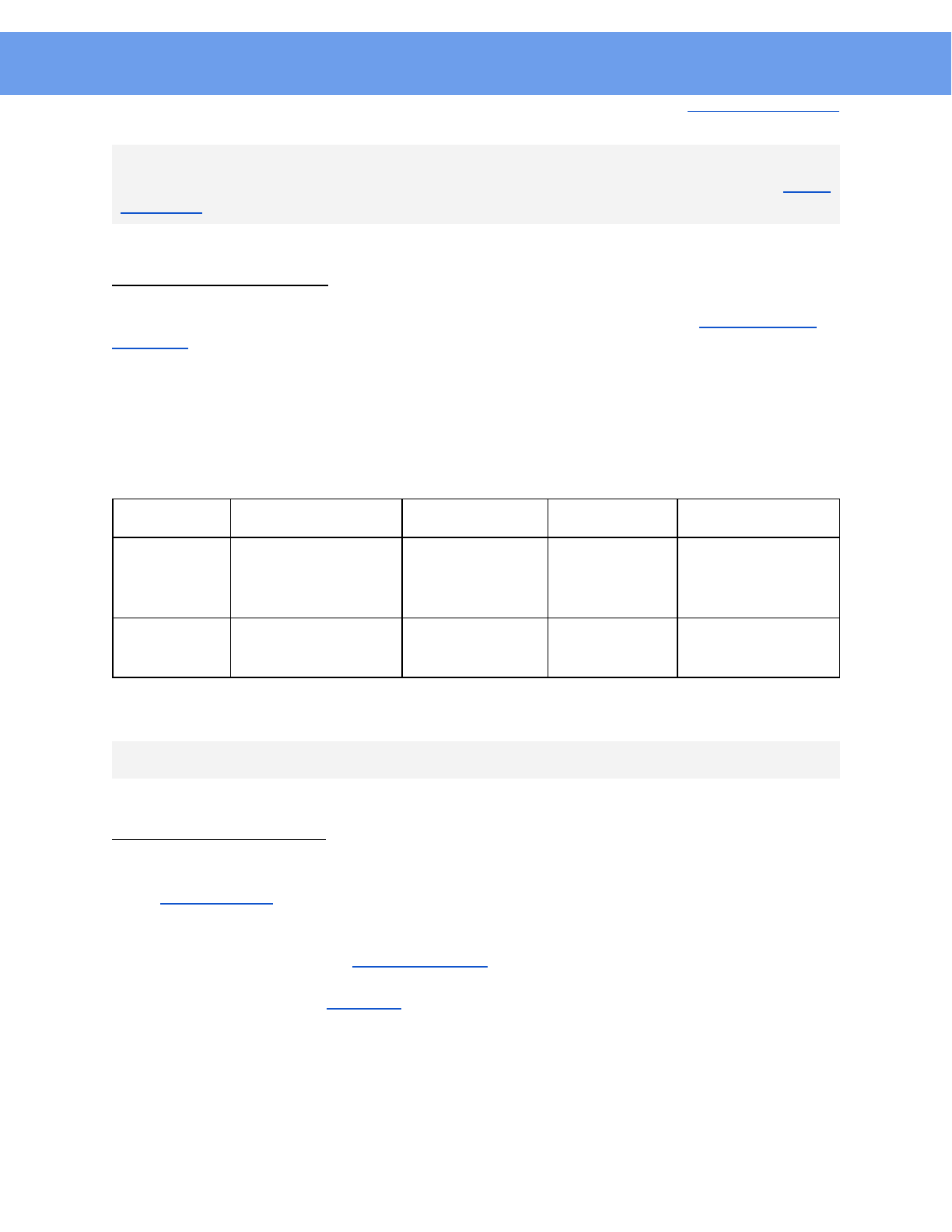
Initial LDA Instructions
A step-by-step guide
Questions:
agasthya.ps@gmail.com
This guide will provide an overview of the strategies employed in the Learner Profile Dashboard
Qualitative Grouping Code as well as step-by-step instructions on how to use it. The code can be
found here.
General Idea and Strategy
In order to make the adaptive engine that powers HPL more robust, we can use Latent Dirichlet
Allocation (LDA) to process students’ qualitative answers (eg essay questions) and use them as
additional data. The LDA model we create can then be used to assign the probability of a particular
student belonging to an interest group based on their answers.
To reduce the amount of time spent on data wrangling, it would be ideal if the data used in this
process is formatted to resemble the following:
Name
Multiple Choice 1
Essay 1
Essay 2
Multiple Choice 2
Student A
Option c
Back when I was
a kid...
What inspires
me most
about...
Option d
Student B
Option b
I used to think
that...
I find that I like
to address...
Option d
That is, each row should represent one student’s answers to every question in the LPD.
Step-by-Step Instructions
This is as specific as possible. However, with each iteration of the course, things will obviously change.
Please
refer to the code
as you read.
Preparing
1. The code in github is in a Jupyter Notebook. This is an “Integrated Development
Environment” (IDE) for Python. The easiest way to install the Jupyter platform on your
computer is to install Anaconda - this is a data science platform that will install Python,
Jupyter, and a host of other applications.
Run the code
1. Read in the data and create a subset that only includes qualitative answers (ie non-multiple
choice).
1
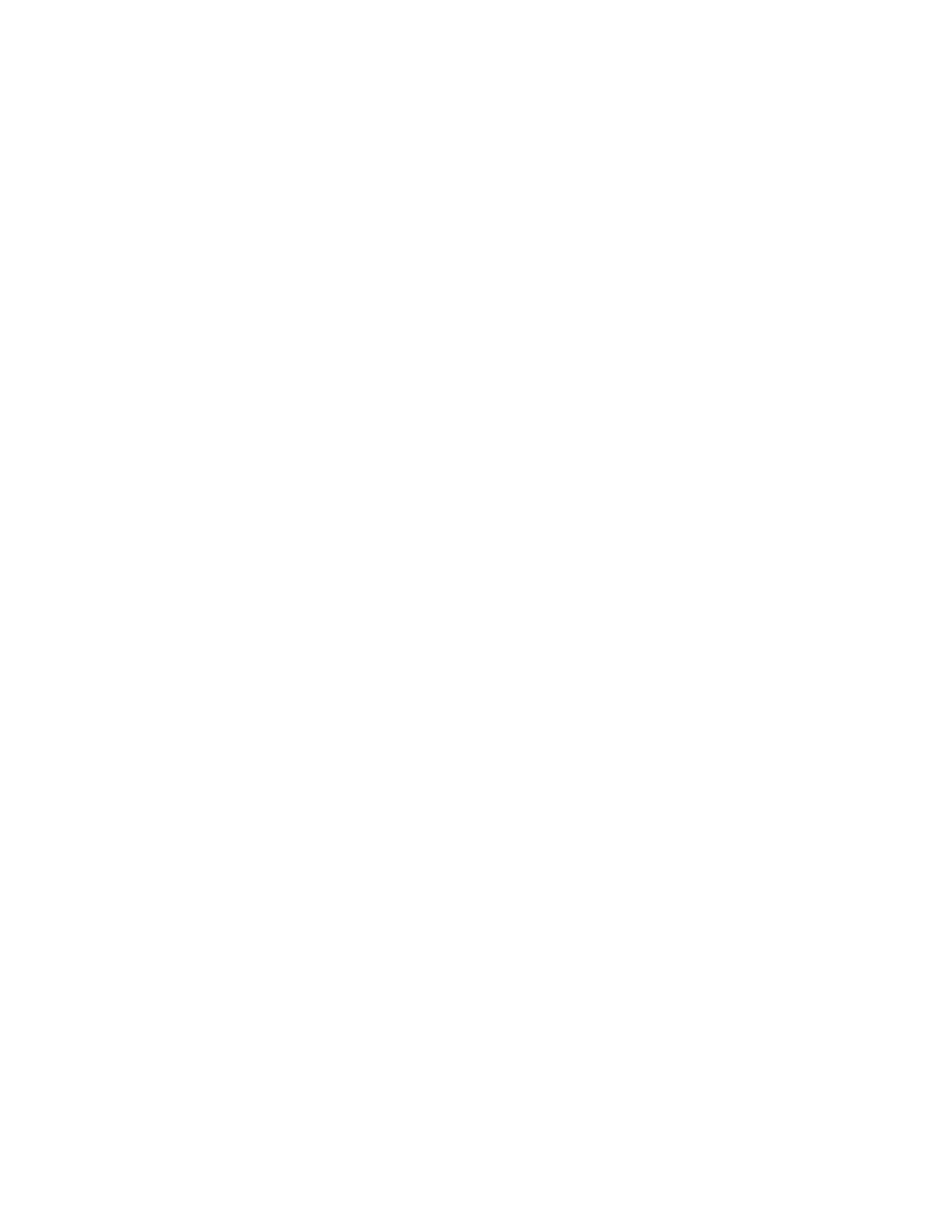
2. Clean student answers by using the cleaning functions.
3. Create a tfidf matrix and fit an untrained LDA model to it.
4. Print out the generated topics:
a. Check for any words that appear too much in each topic.
i. Add those words to the stop word list, re-run step 3.
b. Check for interpretability of topics:
i. If they are difficult to interpret, re-fit the model and print topics until they
are interpretable.
5. Once you have interpretable topics, save the model using the joblib.dump function.
2