Computer Aided Molecular Docking Studies On Diarylsulfonylureas As Potential Anticancer Agents Docker 976 Pxc3894906
User Manual: Docker 976
Open the PDF directly: View PDF .
Page Count: 4

International Journal of Computer Applications (0975 – 8887)
Volume 92 – No.2, April 2014
33
Computer Aided Molecular Docking Studies on
Diarylsulfonylureas as Potential Anticancer Agents
K. L. Deepthi
Asst. Professor
Pharmaceutical Technology
Division
S.V. College of Pharmacy
Etcherla, Srikakulam, India
K. E. V. Nagoji
Professor
Pharmaceutical Analysis
Division
S.V. College of Pharmacy
Etcherla, Srikakulam, India
A. Vasudeva Rao, Ph.D
Assoc. Professor
Pharmaceutical Chemistry
Division
S.V. College of Pharmacy
Etcherla, Srikakulam, India
ABSTRACT
Molecular docking study was performed on a series of 28
Diarylsulfonylureas LD1-LD28 as potential cyclin-dependent
kinase 2 (CDK2) inhibitors. The docking technique was
applied to dock a set of representative compounds within the
active site region of 3PY1 using Molegro Virtual Docker v
5.0. For these compounds, the binding free energy (kcal/mol)
was determined. The docking simulation clearly predicted the
binding mode that is nearly similar to the crystallographic
binding mode with 1.02Ao RMSD. Based on the validations
and hydrogen bond interactions made by R substituents were
considered for evaluation. The results avail to understand the
type of interactions that occur between diarylsulfonylureas
with 3PY1 binding site region and explain the importance of
R substitution on diarylsulfonylurea basic nucleus.
Keywords
Molecular Docking, Diarylsulfonylureas, Cyclin-dependent
kinase 2 (CDK2), Molegro Virtual Docker (MVD).
1. INTRODUCTION
Computer aided drug design (CADD) can be done in two
ways: ligand-based or structure-based. With the availability of
the 3D structure of a biological target, it is feasible to use a
structure-based approach to evaluate and predict the binding
mode of a ligand within the active site of the receptor with
docking methods [1-8]. Now it is a popular technique used for
increasing the speed of drug designing process. This was
made possible by the availability of many protein structures
which helped in developing tools to understand the structure
function relationships, automated docking and virtual
screening.
Cancer is characterized by alterations in the expression of
multiple genes, leading to dysregulation of the normal cellular
program for cell division and cell differentiation. This results
in an imbalance of cell replication and cell death that favours
growth of a tumor cell population. The characteristics that
delineate a malignant cancer from a benign tumor are the
abilities to invade locally, to spread to regional lymph nodes,
and to metastasize to distant organs in the body. At the
molecular level, all cancers have several things in common,
which suggests that the ultimate biochemical lesions leading
to malignant transformation and progression can be produced
in an unidentical pattern which is due to alterations in gene
expression. In general, malignant cancers cause significant
morbidity and will be lethal to the host if not treated.
Exceptions to this appear to be latent, indolent cancers that
may remain clinically undetectable (or in situ), allowing the
host to have a standard life expectancy. Clinically, cancer
appears to posses different phenotypic characteristics. As
cancerous growth progresses, genetic drift in the cell
population produces cell heterogeneity such as cell
antigenicity, invasiveness, and as well metastatic potentials
[9-12].
Cyclin-dependent kinases are the key regulators of cell-cycle
transitions. In mammalian cells, Cdk2, Cdk4, Cdk6 and
associated cyclins control the G1 to S phase transition.
Because proper regulation of this transition is critical for an
organism’s survival, these protein kinases are exquisitely
regulated at different mechanistic levels and in response to a
large variety of intrinsic and extrinsic signals. Cyclin-
dependent kinase 2 (CDK2) in complex with cyclins E and/or
A is a key cell cycle regulator and continues to be an
attractive target for the discovery of new anti-tumor agents. In
particular, inhibitors of CDK-2/cyclin A/E have already
progressed into clinical trials with encouraging early results
[13, 14]. The X-ray crystal structure was obtained from the
Brookhaven Protein Data Bank (http://www.rcsb.org/pdb)
(PDB.ID: 3PY1).
In India cancer is one of the ten leading causes of death today
and advancing in rank year by year. According to the Indian
Cancer Society, about 1.5 million people suffer from cancer at
any point of time in India and India has the world’s highest
incidences of cancer of the gall bladder, mouth and lower
pharynx. In view of present scenario, development of drugs
with target specific predefined anticancer potential is more
essential to fight against various types of cancers. Recently,
the CDK2 inhibitory activity has been hypothesized to possess
therapeutic potential for treatment of cancer. Thus there is a
need for rapid and efficient computational methods capable of
differentiating compounds with acceptable biopharmaceutical
properties, e.g. solubility, lipophilicity, ionization constant etc
at an early stage in the drug discovery process. In the present
study, Ligand Protein Inverse Docking (LPID) stratagies were
employed on set of 28 diarylsulfonylureas which earlier
reported as potential cytotoxic agents. Through In Silico
docking procedures different modes of interactions exhibited
by these ligands will be recognized and further examined for
their predicted binding energies.
2. MATERIALS AND METHODS
2.1 Software Methodology
In the present molecular docking study, software Molegro
Virtual Docker (MVD) v 5.0 (www.molegro.com) along with
Graphical User Interface (GUI), MVD tools was utilized to
generate grid, calculate dock score and evaluate conformers.
Molecular docking was performed using MolDock docking
engine of software. The scoring function used by MolDock is
derived from the Piecewise Linear Potential (PLP) scoring
functions. The active binding site region was defined as a
spherical region which encompasses all protein within 15.0 Ao
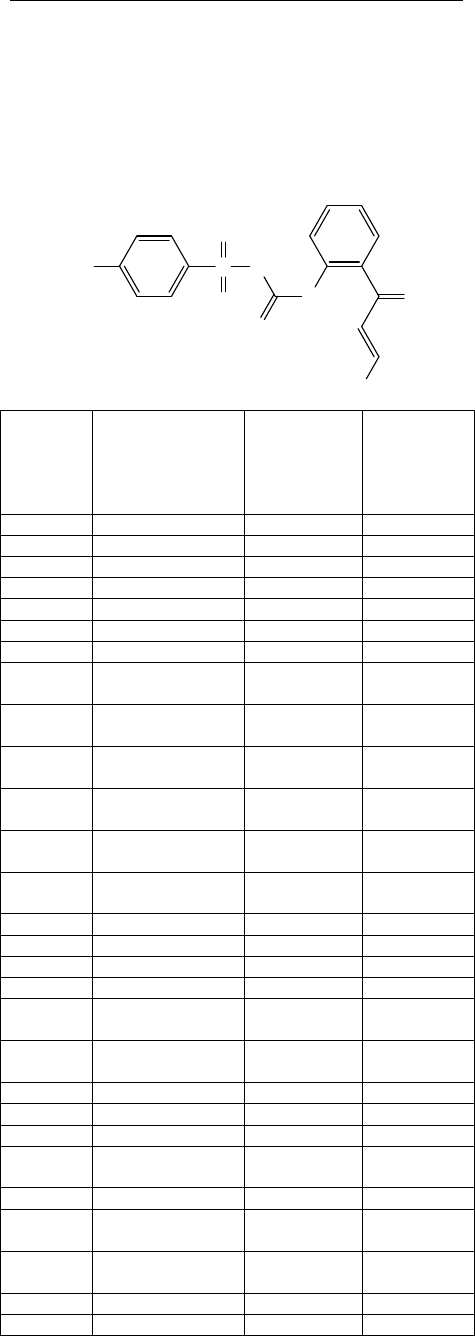
International Journal of Computer Applications (0975 – 8887)
Volume 92 – No.2, April 2014
34
of bound crystallographic ligand atom with selected co-
ordinates of X, Y and Z axes, respectively. Default settings
were used for all the calculations. Docking was performed
using a grid resolution of 0.30 Ao and for each of the 10
independent runs; a maximum number of 1500 iterations were
executed on a single population of 50 individuals. The active
binding site was considered as a rigid molecule, whereas the
ligands were treated as being flexible, i.e. all non-ring torsions
were allowed [15].
2.2 Molecular Modeling
A set of 28 new diarylsulfonylureas LD1-LD28 listed in
Table 1, were synthesized, characterized and which earlier
reported as potential cytotoxic agents by one of the authors
Dr. Vasudeva Rao Avupati et al [16]. In the present study, a
set of 28 new diarylsulfonylureas LD1-LD28 were modeled
by using ISIS DRAW 2.2 software.
2.3 Ligand Preparation
The structures of diarylsulfonylureas LD1-LD28 were
converted into suitable chemical information using Chemdraw
ultra v 10.0 (Cambridge software), copied to Chem3D ultra v
10.0 to create a 3D model and, finally subjected to energy
minimization using molecular mechanics (MM2). The
minimization was executed until the root mean square
gradient value reached a value smaller than 0.001kcal/mol.
Such energy minimized structures are considered for docking
and corresponding pdb files were prepared using Chem3D
ultra v 10.0 integral option (save as /Protein Data Bank (pdb))
(Table 1) [17].
2.4 Protein Selection
The selection of protein for docking studies is based upon
several factors i.e. structure should be determined by X-ray
diffraction, and resolution should be between 2.0-2.5Ao, it
should contain a co-crystallized ligand; the selected protein
should not have any protein breaks in their 3D structure.
However, we considered ramachandran plot statistics as the
important filter for protein selection that none of the residues
present in disallowed regions [18].
2.5 Protein Preparation
All CDK2 X-ray crystal structures were obtained from the
Brookhaven Protein Data Bank (http://www.rcsb.org/pdb).
Subsequent to screening for the above specific standards the
resultant protein target (PDB Code: 3PY1) was selected and
prepared for molecular docking simulation in such a way that
all heteroatoms (i.e., nonreceptor atoms such as water, ions,
etc.) were removed and Kollmann charges were assigned [19].
2.6 Software Method Validation
Software method validation was performed in MVD using
Protein Data Bank (PDB) protein 3PY1. The x-ray crystal
structure of 3PY1 complex with co-crystallized ligand was
recovered from PDB. The bio active co-crystallized bound
ligand was docked with in the active site region of 3PY1. The
RMSD of all atoms between the two conformations is 1.02 Ao
indicating that the parameters for docking simulation are good
in reproducing X-ray crystal structure.
2.7 Molecular Docking
In the present investigation, we make use of a docking
algorithm called MolDock. MolDock is based on a new
hybrid search algorithm, called guided differential evolution.
The guided differential evolution algorithm combines the
differential evolution optimization technique with a cavity
prediction algorithm. We used MVD because it showed
higher docking accuracy than other stages of the docking
products (MVD: 87%, Glide: 82%, Surflex: 75%, FlexX:
58%) in the market [20, 21].
Table 1. Diarylsulfonylureas LD1-LD28 with their
Moldock Scores (kcal/mol) and H-bonds interactions
against Cyclin-dependent kinase 2 (CDK2)
H3C S
O
O
NH
O
NH
R
O
Ligand
Code
‘R’ Group
Substituent
Moldock
Score
(kcal/mol)
No. of H-
Bonds /
H-bond
Interacting
Residues
LD1
C6H5
-142.793
1/Leu 66
LD2
4-MeC6H4
-148.509
1/Lys 134
LD3
4-NMe2C6H4
-147.976
1/Ser 283
LD4
3-OMeC6H4
-154.771
1/Arg 509
LD5
4-OMeC6H4
-146.259
1/Tyr 308
LD6
3,4-diOMeC6H3
-154.008
Nil
LD7
2,4-diOMeC6H3
-154.88
Nil
LD8
3,4,5-tri
OMeC6H2
-152.219
1/Cys 29
LD9
2-OHC6H4
-144.888
2/Gly 104,
His 151
LD10
3-OHC6H4
-151.099
2/Glu 211,
Asp 274
LD11
4-OHC6H4
-147.838
2/Arg 506,
Trp 450
LD12
3-OEt,4-OHC6H3
-147.356
2/Glu 208,
Phe 210
LD13
3-OMe,4-
OHC6H3
-141.17
1/Met 74
LD14
2-NO2C6H4
-131.464
Nil
LD15
3-NO2C6H4
-148.493
Nil
LD16
5-OH,2-NO2C6H3
-148.088
1/Asp 274
LD17
3-FC6H4
-154.175
Nil
LD18
4-FC6H4
-151.071
2/Glu 211,
Asp 274
LD19
2-ClC6H4
-214.426
3/Phe 146,
Val 64
LD20
4-ClC6H4
-151.658
1/His 183
LD21
2,4-diClC6H3
-141.447
1/Tyr 67
LD22
3-BrC6H4
-146.84
1/Glu 122
LD23
4-Allyl-OC6H4
-160.316
2/Lys 721,
Asp 831
LD24
Phenylethene-yl
-184.534
1/Arg 132
LD25
Pyrrol-2-yl
-156.15
2/Phe 146,
Val 64
LD26
Pyridin-3-yl
-139.734
2/Ser 148,
Lys 147
LD27
Pyridin-4-yl
-141.422
1/Glu 73
LD28
Anthracen-9-yl
-165.854
1/Tyr 67
Molecular docking technique was employed to dock the
designed diarylsulfonylureas LD1-LD28 listed in (Table 1)
against CDK2 receptor 3PY1 using MVD to locate the
interaction between various compounds and CDK2. MVD
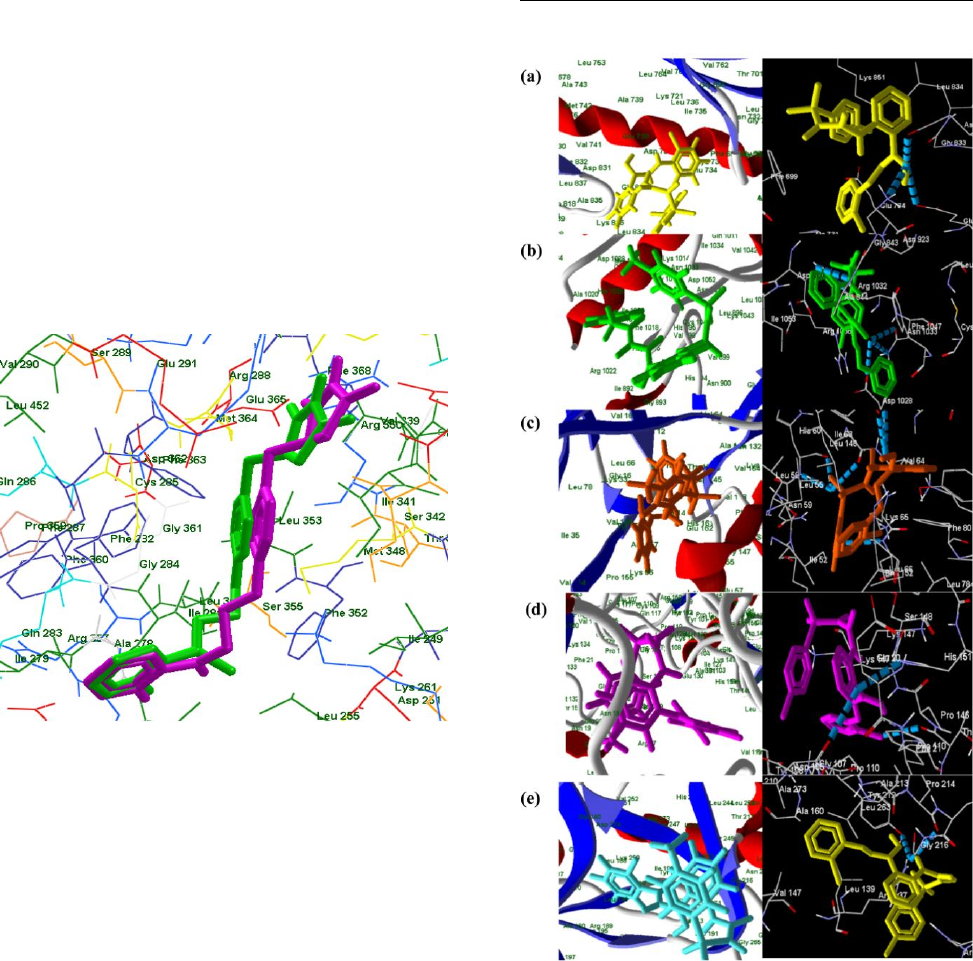
International Journal of Computer Applications (0975 – 8887)
Volume 92 – No.2, April 2014
35
requires the receptor and ligand coordinates in either Mol2 or
PDB format. Non polar hydrogen atoms were removed from
the receptor file and their partial charges were added to the
corresponding carbon atoms. Molecular docking was
performed using MolDock docking engine of Molegro
software. The binding site was defined as a spherical region
which encompasses all protein atoms within 15.0 Ao of bound
crystallographic ligand atom (dimensions X (22.34 A°), Y (-
83.32 A°), Z (-22.11 A°) axes, respectively). Default settings
were used for all the calculations. Docking was performed
using a grid resolution of 0.3 Ao and for each of the 10
independent runs; a maximum number of 1500 iterations were
executed on a single population of 50 individuals.
Fig 1: Superimposed binding orientation of docked
conformer (pink) and most stable ligand (green) within the
active binding site region of 3PY1.
3. RESULTS AND DISCUSSION
Ligand-Protein Inverse Docking (LPID) approach has been
used as a useful tool in facilitating drug design. In this
approach, docking single or multiple small molecules in
single or multiple conformations to a receptor site is
attempted to find putative ligands. A number of flexible
docking algorithms have been introduced. These include
multiple-conformer shape matching, genetic algorithm,
evolutionary programming, simulated annealing, fragment-
based docking, and other novel algorithms. Testing results
have shown that these algorithms are capable of finding
ligands and binding conformations at a receptor site close to
experimentally determined structures. Because of their
capability in identifying potential ligands and binding
conformations, these algorithms are expected to be equally
applicable to an inverse-docking process for finding multiple
putative protein targets to which a small molecule can bind or
weakly bind. This may be applied to the identification of
unknown and secondary therapeutic targets of drugs, drug
leads, natural products and other ligands. LPID approach is
now applied to the database of 28 compounds in the present
study for finding possible binding orientation, binding mode
and binding interaction within the active site region of CDK2.
The compound with least binding energy against target
protein is considered as ‘hit compound’. By this means, it is
possible to understand how the compounds with observed
cytotoxicity interact with the target protein. The results
emerging out of this study can be used to establish the
possible inherent mechanism of action of diarylsulfonylureas
as potential cytotoxic agents.
Fig 2. a) Active binding mode and H-bond interactions of
LD19 against CDK-2 b) Active binding mode and H-bond
interactions of LD23 against CDK-2 c) Active binding
mode and H-bond interactions of LD24 against CDK-2 d)
Active binding mode and H-bond interactions of LD25
against CDK-2 e) Active binding mode and H-bond
interactions of LD28 against CDK-2
The ligand-protein inverse docking simulation technique was
performed using MVD program with 28 synthetic ligands
diarylsulfonylureas LD1-LD28 with basic α,β-unsaturated
ketone and sulfonylurea moieties reported to be having
Cyclin-dependent kinase 2 (CDK2) inhibitory activity.
Docking simulations with 3PY1 bound ligand resulted in a
Moldock score of -128.38 kcal/mol and a RMSD value of
1.72 Ao showed no hydrogen bond interactions with in the
active binding site region. Docking studies on experimental
compounds (Table 1) showed that most stable binding ligand
LD19 involved in 3 hydrogen bonds with amino acid residues
Phe 146 and Val 64 within the binding site region of 3PY1.
Although, other H-bond interactions exist, these hydrogen
bonds are relevant for inducing intrinsic activity towards
highly selective and CDK2 specific inhibitory property.
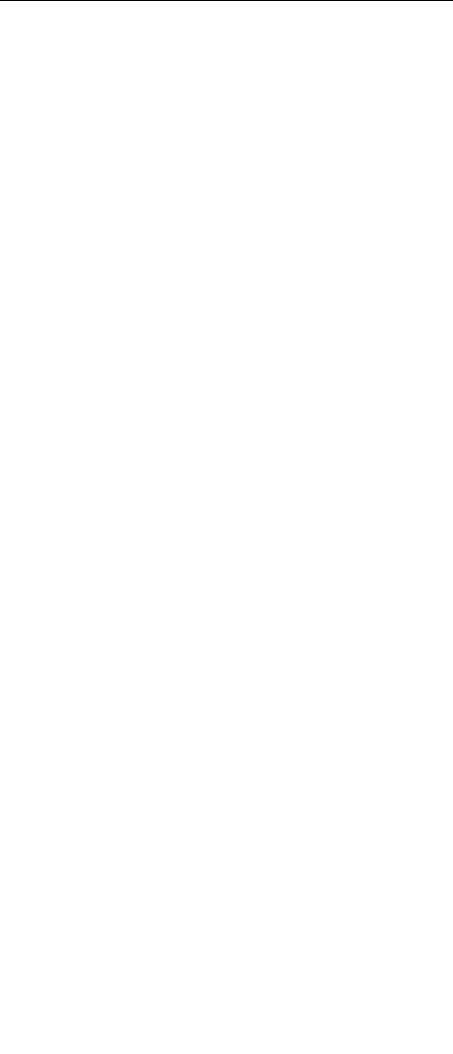
International Journal of Computer Applications (0975 – 8887)
Volume 92 – No.2, April 2014
36
Moreover, from the data given in (Table 1), it appears that the
compound LD19 represent most significant among the diverse
range of compounds. The amino acids Phe 146 and Val 64
were appeared to be the most important binding site residues
that participate in H-bond interactions with in the active
binding site region of 3PY1. The noteworthy hypothesis
recognized by our studies on experimental compounds is
useful in predicting the key interacting ligand LD19 and its
binding properties to exhibit CDK2 specific inhibitory
property. Among all the compounds with stable binding
conformations as seen in case of compounds such as LD19,
LD23, LD24, LD25 and LD28 and LD17 with Moldock
Score i.e. least binding energies with corresponding H-bonds
and interacting residues -214.426 kcal/mol, 3/Phe 146, Val 64,
-160.316 kcal/mol, 2/Lys 721, Asp 831, -184.534 kcal/mol,
1/Arg 132, -156.15 kcal/mol, 2/Phe 146, Val 64 and -165.854
kcal/mol, 1/Tyr 67 respectively (Fig 2)
4. CONCLUSIONS
In this study the ligand-protein molecular docking simulation
was used to preliminarily investigate and to confirm the
potential molecular target for the diarylsulfonylureas LD1-
LD28 with observed cytotoxicity. The analysis of the best
docked ligands against selected anticancer drug target
revealed the binding mode of compounds involved in this
study and confirm the role as CDK2 inhibitors. Binding
energies of the drug–enzyme (receptor) interactions are
important to describe how fit the drug binds to the target
macromolecule. The residues participated in the hydrogen
bond formation within the active binding site region revealed
the importance of these residues towards the observed binding
energy with respect to the hit identified against CDK2 target
protein. The obtained hypothesis could be the remarkable
starting point to develop some new leads as potential CDK2
inhibitors with enhance the affinity as well as intrinsic
activity. The results of this work indicate efficient
computational tools are capable of identify potential ligands
such as LD19, LD23, LD24, LD25 and LD28 which earlier
reported in our work as potential cytotoxic agents.
5. ACKNOWLEDGMENTS
One of the authors Dr. Vasudeva Rao Avupati is thankful to
M/S Molegro Aps, Denmark for providing software license
during the course of our research work.
6. REFERENCES
[1]. Drews, J. (2000) Drug discovery: A historical
Perspective. Scinece 287, 1960-1964.
[2]. Ohlstein, EH., Ruffolo Jr., R.R., and Ellroff, J.D. (2000)
Drug discovery in the next millennium. Annu. Rev.
Pharmacol. Toxicol. 40, 177-191.
[3]. Royer RJ. Mechanism of action of adverse drug
reactions: An overview. 1997; Pharmcoepidemiology
and drug safety 6 (Suppl.):S43-S50.
[4]. DiMasi JA, Bryant, NR, Lasagna L. (1991). New drug
development in the United States from 1963 to 1990.
Clin Pharmacol Ther. 50, 471-486.
[5]. Chen Y.Z., and Zhi D. G., (2001). Ligand-Protein
Inverse Docking and Its Potential Use in Computer
Search of Putative Protein Targets of a Small Molecule.
Proteins, 43, 217-226
[6]. Chen Y.Z., and Ung C. Y. (2002). Computer Automated
Prediction of Putative Therapeutic and Toxicity Protein
Targets of Bioactive Compounds from Chinese
Medicinal Plants, Am. J. Chin. Med., 30, 139-154.
(2002).
[7]. Chen Y.Z., and Ung C. Y. (2001). Prediction of Potential
Toxicity and Side Effect Protein Targets of a Small
Molecule by a Ligand-Protein Inverse Docking
Approach. J. Mol. Graph. Mod., 20, 199-218.
[8]. Cornell, WD, Cieplak P, Bayly CI, Gould IR, Merz KM
Jr, Ferguson DM, Spellmeyer DC, Fox T, Caldwell JW,
Kollman PA. A second generation force field for the
simulation of proteins and nucleic acids. J. Am. Chem.
Soc. 1995; 117:5179-5197.
[9]. Lorber DM, Shoichet BK. Flexible ligand docking using
conformational ensembles. Protein Sci. 7:938-950. 1998.
[10]. Schmidt TJ, Meyer AS. Autoregulation of corticosteroid
receptors. How, when, where, and why? Receptor. 1994
Winter;4:229-257.
[11]. Sandak B, Wolfson HJ, Nussinov R. Flexible docking
allowing induced fit in proteins: insights from an open to
closed conformational isomers. Proteins 1998;32:159-
174.
[12]. Noreen Y, Serrano G, Perera P, Bohlin L. Flavan-3-ols
isolated from some medicinal plants inhibiting COX-1
and COX-2 catalysed prostaglandin biosynthesis. Planta
Med. 64:520-524. 1998.
[13]. Sausville, E. A. Curr. Med. Chem. (2003), 3, 47.
[14]. Fischer, P. M.; Gianella, B. A. Exp. Opin. Invest. Drugs.
(2003), 12, 955.
[15]. Gehlhaar, D. K.; Verkhivker, G.; Rejto, P. A.; Fogel, D.
B.; Fogel, L. J.; Freer, S. T. (1995) Docking
Conformationally Flexible Small Molecules Into a
Protein Binding Site Through Evolutionary
Programming. Proceedings of the Fourth International
Conference on Evolutionary Programming, No 123-124.
[16]. Vasudeva Rao Avupati, Rajendra Prasad Yejella, Girija
Sankar Guntuku, Pradeepsagar Gunta (2012) Synthesis,
characterization and biological evaluation of some novel
diarlsulfonylureas as potential cytotoxic and
antimicrobial agents. Bioorganic & Medicinal Chemistry
Letters, 22, 1031–1035.
[17]. Berman H M, Westbrook J, Feng Z, Gilliland G, Bhat T
N, Weissig H, Shindyalov I N, Bourne P E (2000) The
Protein Data Bank. Nucleic Acids Research, 28, 235-
242.
[18]. Wang, J, Kollman, PA, Kuntz, ID. Flexible ligand
docking: A multistep strategy approach. Proteins. 1999;
36:1-19.Bowman, M., Debray, S. K., and Peterson, L. L.
1993. Reasoning about naming systems.
[19]. Ramachandran, G. N., Sasisekharan, V. (1968)
Conformation of polypeptides and proteins. Adv. Protein
Chem. 23, 283-438.
[20]. Vasudeva Rao Avupati, Purna Nagasree Kurre, Santoshi
Rupa Bagadi, Muralikrishna Kumar Muthyala and
Rajendra Prasad Yejella (2010) Denovo Based Ligand
generation and Docking studies of PPARδ Agonists.
Correlations between Predicted Biological activity LD.
Biopharmaceutical Descriptors. 10, 74-86.
[21]. Storn, R., Price, K. Differential Evolution - A Simple and
Efficient Adaptive Scheme for Global Optimization over
Continuous Spaces. Tech-report, International Computer
Science Institute, Berkley, 1995.
IJCATM : www.ijcaonline.org